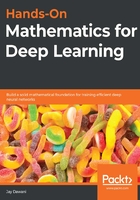
Independence
Events are independent when they are not related to each other; that is, the outcome of one has no bearing on the outcome of another.
Suppose we have two independent events, A and B. Then, we can test the following:

If this is not true, then the events are dependent.
Imagine you're at a casino and you're playing craps. You throw two dice—their outcomes are independent of each other.
An interesting property of independence is that if A and B are independent events, then so are A and BC.
Let's take a look and see how this works:

When we have multiple events, A1, A2, …, An, we call them mutually independent when for all cases of n ≥ 2.
Let's suppose we conduct two experiments in a lab; we model them independently as and
and the probabilities of each are
and
, respectively. If the two are independent, then we have the following:

This is for all cases of i and j, and our new sample space is Ω = Ω1 × Ω2.
Now, say A and B are events in the Ω1 and Ω2 experiments, respectively. We can view them as subspaces of the new sample space, Ω, by calculating A × Ω2 and B × Ω1, which leads to the following:

Even though we normally define independence as different (unrelated) results in the same experiment, we can extend this to an arbitrary number of independent experiments as well.