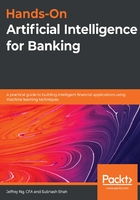
Importance of accessible banking
Like electricity and water, banking should be made as widely and easily available as utilities. Only when we make banks efficient can we make them accessible and have them benefit the highest number of people possible. Banking is a service that is provided to make the best use of capital/money to generate returns for those whosaveand/orthose who need the capital to have a more productive life at an agreed risk and return.
What we want to do is to be consistent with Robert J. Shiller's sentiment in his book,Finance and the Good Society, where he indicates the necessity of information technology in finance to help achieve our goals. A step further would be to leverage open source methods and applications to solve the accessibility challenges of the banking industry. Open source software solutions tend to be cost-effective, robust, and secure.
To make banking accessible, one of the most important things to do is to have a lot of data. This will make decisions more efficient and transparent, which can help to reduce the cost of banking decisions. We will discuss the need for open source data in the next section. By virtue of the competitive banking market, the price of banking services will gradually decrease as banks with good efficiency will win a large market share.
Once implemented in the financial sector, AI will have three impacts on the sector—the job of repetitive tasks will be eliminated, there will be increased efficiency with AI augmenting human, and job creation with new AI-related tasks such as model building. Out of these three, job reduction and increased efficiency will impact existing jobs, whereas job creation will have an impact on future talent and the job market.
As automation and efficiency increase, existing jobs will be altered and impacted. Machines will perform day-to-day tasks with more efficiency than humans can. However, to manage, monitor, maintain, and enhance tasks performed by machines or AI, the industry will become open to skilled, techno-functional professionals who understand both banking and AI technology.
Open source software and data
The speed of technological development in the past 20 years or so has been quite rapid due to the open source movement. It started with Linux and was followed by ImageNet. ImageNet provided lots of training data. This training data fueled the activities of technicians who worked in research on developing AI algorithms. These technicians developed algorithms for deep learning and neural networks using open source libraries written in programming languages such as Python, R, scikit-learn, TensorFlow, and more.
While the open source approach encourages software development, another key ingredient of AI is data. Finding practical open data is a challenge. Banks, on the other hand, have the challenge of converting data into a machine-trainable dataset cautiously and safely to make sure that there is no breach of data that customers entrusted the bank with.
Today, in the finance and banking world, client confidentiality remains a keyobstacleto opening up data for wider research communities. Real-world problems can be more complex than what we have seen in the open data space. Opening up data stored in databases can be a practical step, while opening up images, such as documents, audio files, or voice dialogues, for example, can be challenging as this data, once masked or altered, may lose some information systematically.
In fact, the major cost of implementing real-life applications in banking also comes from the data-feed subscription. The cost of data collection and aggregation is a major challenge that you will see in this book. How our society is handling this problem and incentivizing the commercial sector to tackle it requires further discussion beyond the scope of this book. Following this same spirit, the code for this book is open source.
Why do we need AI if a good banker can do the job?
Let's consider a single financial task of matching demand for capital in the funding markets. This is a highly routine task of matching numbers. Here, it is obvious that the computer would be a better fit for the job.
The goal of employing AI is to make machines do the things that humans do right now, but with more efficiency. Many people wonder whether applying AI in banking might affect the jobs of those working in the industry.
Do remember that the aim is not to replace humans, but to augment the current human capacity to improve productivity, which has been the goal of technology throughout the history of human civilization. Humans are known to be weaker in determining accurate probability, as shown in the psychological research paper, Thinking, Fast and Slow, April 2, 2013, by Daniel Kahneman. Therefore, it is challenging to make a probability decision without a computer.